GLiDE: Generalizable Quadrupedal Locomotion in Diverse Environments with a Centroidal Model
Zhaoming Xie, Xingye Da, Buck Babich, Animesh Garg, Michiel van de Panne
Abstract
Model-free reinforcement learning (RL) for legged locomotion commonly relies on a physics simulator that can
accurately predict the behaviors of every degree of freedom of the robot.
In contrast, approximate reduced-order models are often sufficient for many model-based control strategies.
In this work we explore how RL can be effectively used with a centroidal model to generate robust control policies for quadrupedal locomotion.
Advantages over RL with a full-order model include a simple reward structure, reduced computational costs, and robust sim-to-real transfer.
We further show the potential of the method by demonstrating stepping-stone locomotion, two-legged in-place balance, balance beam locomotion,
and sim-to-real transfer without further adaptations.
Main Video
Acknowledgements
This project is supported by the following.
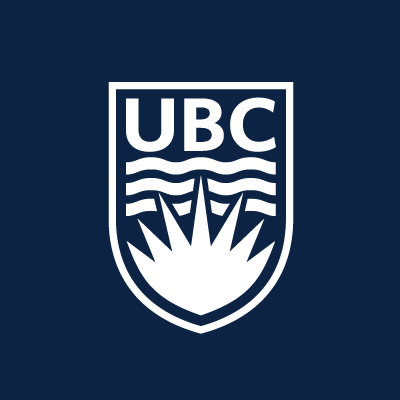
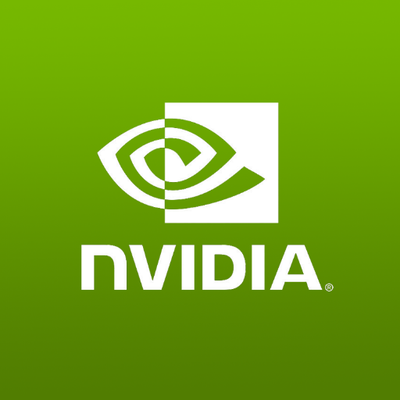
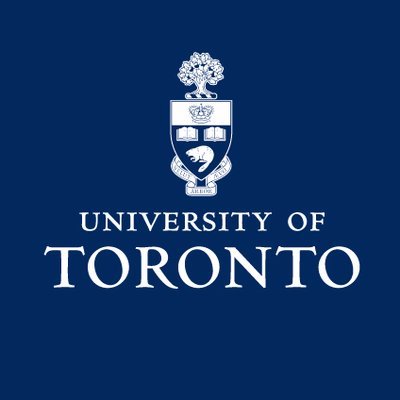
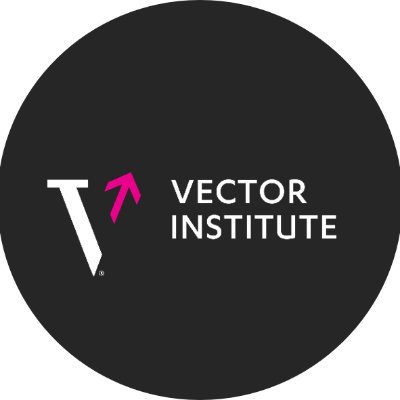